
Given your background as the inventor of hashgraph and your PhD from Carnegie Mellon, how do you envision machine learning algorithms revolutionizing the way we approach blockchain security?
I think there are several ways in which AI can help software security in generally, and so will also help blockchain or DLT security. AI systems can look at source code for smart contracts or for validator nodes to look for bugs or design flaws. This can be done in real time during development (such as during each commit), and can also be used to scan existing code for potential issues.AI systems can also scan traffic, and look for anomalies and signs of potential attacks. On the ledger, this traffic can be the transactions that have been processed on the network. On a particular node, it can be a scan of packets coming in through various ports. In the longer term, I think the future of software security will include "formal methods", where code and protocols have math proofs of their correctness, and the proofs are checked by a computer. Today, it is very difficult and time consuming to build such a proof. But there is research now to see if AI can make the a proof assistant that can make this faster and easier.
With your extensive experience in both national defense and cybersecurity, how do you see machine learning bridging the gap between these fields and blockchain technology?
For national defense and cybersecurity, the formal methods may become so important that all critical software will end up having math proofs checked by computers. And perhaps also compilers, operating systems, and even the computer chips themselves. Research has shown good results at every level of that stack. If AI can make it practical and economical, then it might end up being used for the entire stack.
What are some of the most overlooked vulnerabilities in current cryptographic algorithms, and how can machine learning help identify these weak spots?
There is an enormous range of ways software can have security problems. That's why it isn't enough to just do testing and have multiple people look at the code. There is a real need for stronger, automated analysis of the code. And machine learning may be able to give us systems that can do this. These formal methods are a particularly good fit for machine learning, because it can be based on "reinforcement learning" (trial and error) rather than "supervised learning".
Can machine learning be used to optimize tokenomics in a way that enhances both utility and security within a blockchain ecosystem?
Economics and pricing decisions can be based on models of the economy and of consumer behavior. Supervised can play a role in automatically building such models, if there is enough data. Once the models have been built, reinforcement learning can be used to find optimal actions to optimize the outcomes.
What drives you to integrate machine learning into the blockchain space, and how do you think this integration could redefine the concept of digital trust?
Trust in blockchain is strongly influenced by whether the ledger has secure code. And whether the smart contracts and apps built on top of it have secure code. And whether wallet sofware and other tools have secure code. Strong guarantees of security typically require extreme costs, but there is true potential for machine learning to enable much higher security for reasonable effort and cost.
Key Talks: Interview with Dr. Leemon Baird
The integration of machine learning into blockchain technology and its potential impact on security, national defense, and cryptographic vulnerabilities.


Co-CEO of SwirldsLabs, Co-Founder of Hedera, Inventor of Hashgraph
Given your background as the inventor of hashgraph and your PhD from Carnegie Mellon, how do you envision machine learning algorithms revolutionizing the way we approach blockchain security?
I think there are several ways in which AI can help software security in generally, and so will also help blockchain or DLT security. AI systems can look at source code for smart contracts or for validator nodes to look for bugs or design flaws. This can be done in real time during development (such as during each commit), and can also be used to scan existing code for potential issues.AI systems can also scan traffic, and look for anomalies and signs of potential attacks. On the ledger, this traffic can be the transactions that have been processed on the network. On a particular node, it can be a scan of packets coming in through various ports. In the longer term, I think the future of software security will include "formal methods", where code and protocols have math proofs of their correctness, and the proofs are checked by a computer. Today, it is very difficult and time consuming to build such a proof. But there is research now to see if AI can make the a proof assistant that can make this faster and easier.
With your extensive experience in both national defense and cybersecurity, how do you see machine learning bridging the gap between these fields and blockchain technology?
For national defense and cybersecurity, the formal methods may become so important that all critical software will end up having math proofs checked by computers. And perhaps also compilers, operating systems, and even the computer chips themselves. Research has shown good results at every level of that stack. If AI can make it practical and economical, then it might end up being used for the entire stack.
What are some of the most overlooked vulnerabilities in current cryptographic algorithms, and how can machine learning help identify these weak spots?
There is an enormous range of ways software can have security problems. That's why it isn't enough to just do testing and have multiple people look at the code. There is a real need for stronger, automated analysis of the code. And machine learning may be able to give us systems that can do this. These formal methods are a particularly good fit for machine learning, because it can be based on "reinforcement learning" (trial and error) rather than "supervised learning".
Can machine learning be used to optimize tokenomics in a way that enhances both utility and security within a blockchain ecosystem?
Economics and pricing decisions can be based on models of the economy and of consumer behavior. Supervised can play a role in automatically building such models, if there is enough data. Once the models have been built, reinforcement learning can be used to find optimal actions to optimize the outcomes.
What drives you to integrate machine learning into the blockchain space, and how do you think this integration could redefine the concept of digital trust?
Trust in blockchain is strongly influenced by whether the ledger has secure code. And whether the smart contracts and apps built on top of it have secure code. And whether wallet sofware and other tools have secure code. Strong guarantees of security typically require extreme costs, but there is true potential for machine learning to enable much higher security for reasonable effort and cost.
One Bank
for all digital assets
Manage your assets through one interface via a browser or an app with unparalleled convenience.
Sign Up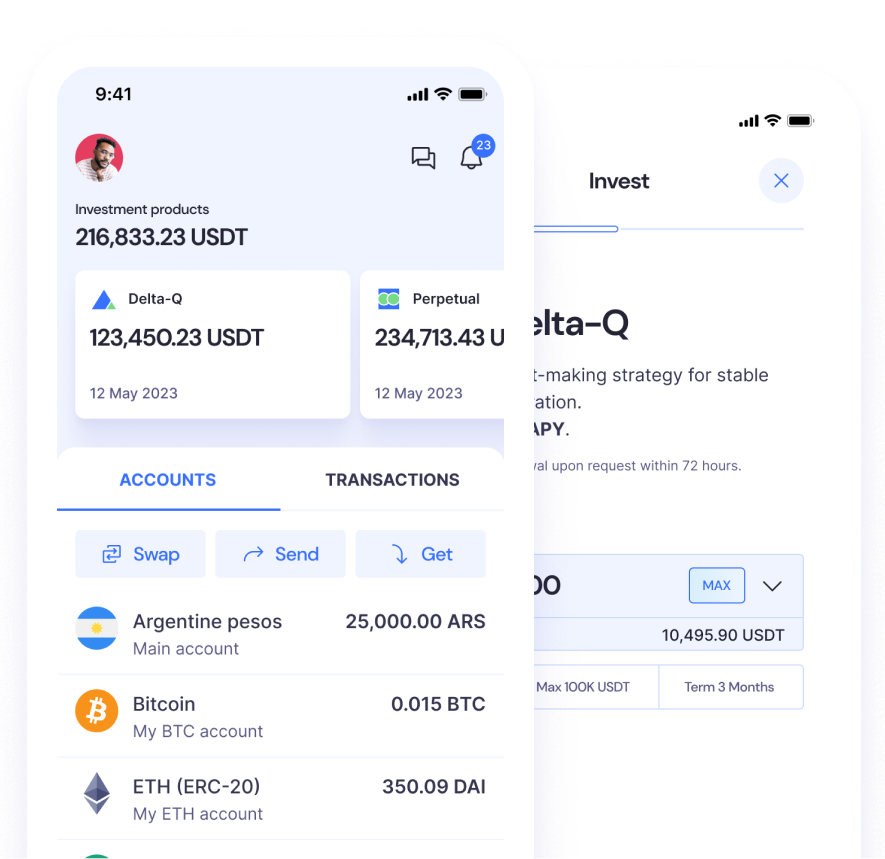
